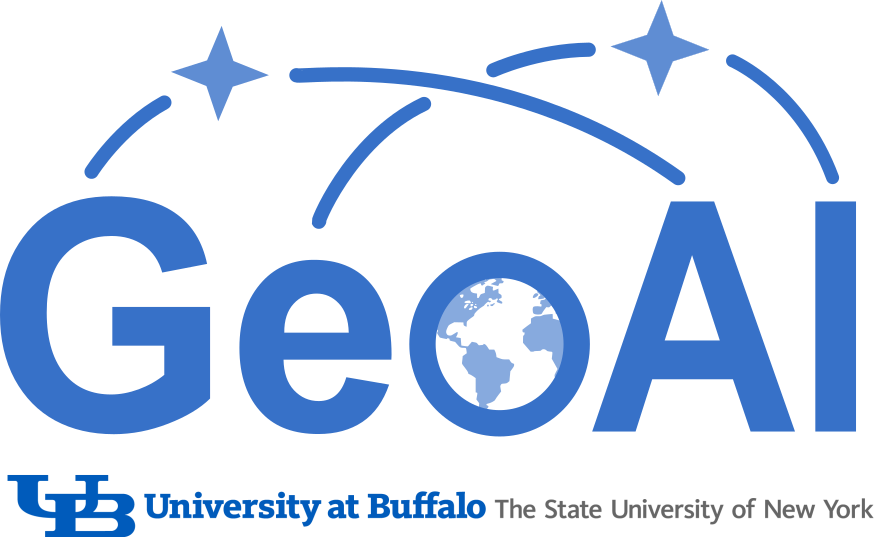
Welcome to the UB Geospatial Artificial Intelligence (GeoAI) Lab! We are a research group located within the Department of Geography at the University at Buffalo (UB). We are also an affiliated lab with the UB AI and Data Science Institute. We conduct basic research that integrates geospatial big data, AI methods, and spatial analysis to study human-environment interactions and to address some of the challenging problems facing our society, such as those related to disaster response and community resilience, public health and environment, and ecosystems and biodiversity. Such basic research also inspires us to conduct methodological research that improves GIS and GeoAI methods, which can further support scientific discoveries.
Our research currently focuses on four interrelated areas:
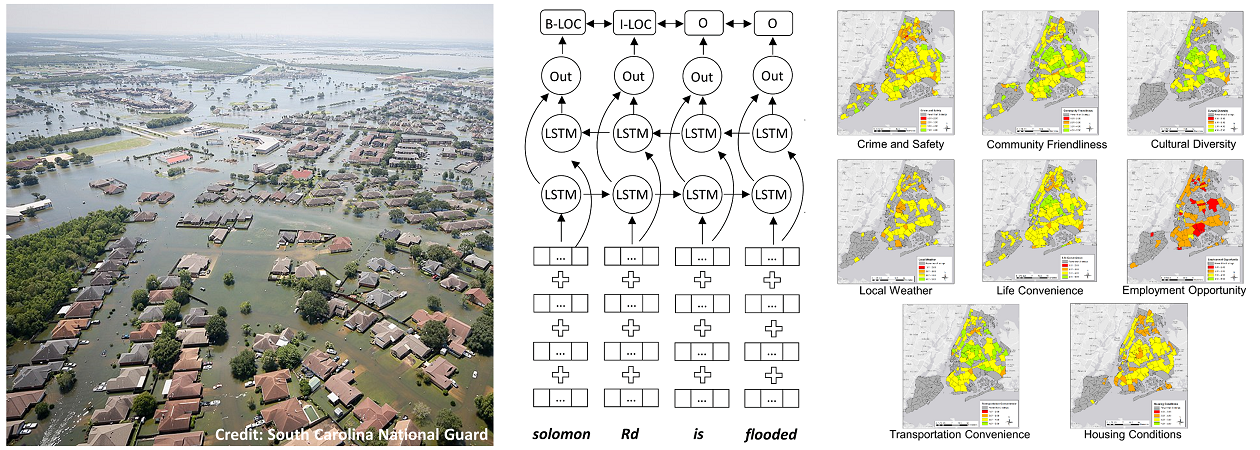
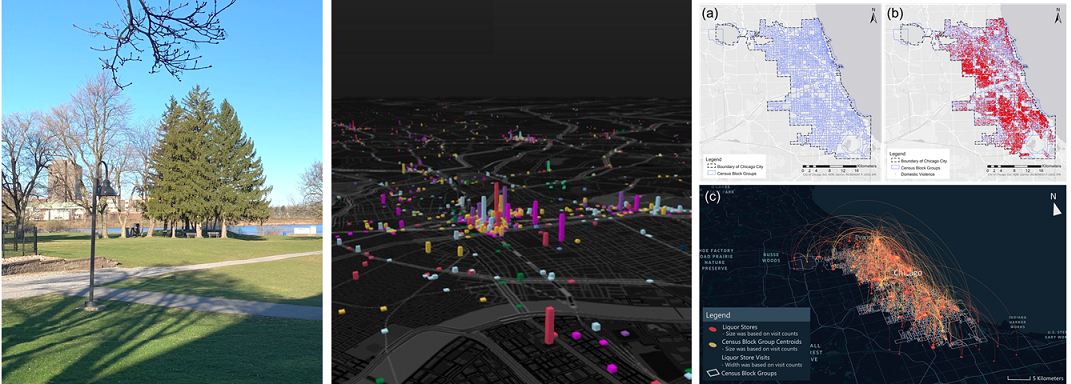
Ecosystems and Biodiversity: Ecosystems and biodiversity provide critical resources for our humans to survive and prosper, which include oxygen, food, clean air and water, pest control, and many others. Our research in this area integrates AI methods, geospatial big data (e.g., remote sensing images and field observations), and spatial models to discover knowledge that advances our understanding of spatial ecological processes (e.g., vegetation dynamics) and informs decisions related to environmental protection and resource management. Our work currently focuses on the Cape Floristic Region of South Africa, which is a global biodiversity hotspot and a UNESCO World Heritage site.
GIS and GeoAI Methods: Basic research in the above three areas inspires us to develop and improve GIS and GeoAI methods and to enhance spatial data infrastructures (SDIs). Improved GIS and GeoAI methods can help us better analyze geospatial data, and enhanced SDIs can facilitate the reuse of existing datasets, tools, and services. Our research in this area has developed methods for prioritizing disaster mapping tasks, enriching the metadata of geospatial resources, and building knowledge graphs from historical maps. We are also one of the participants that developed the five-star guide for achieving replicability and reproducibility in GIScience research.