Abstract: Geographical random forest (GRF) is a recently developed and spatially explicit machine learning model. With the ability to provide more accurate predictions and local interpretations, GRF has already been used in many studies. The current GRF model, however, has limitations in its determination of the local model weight and bandwidth hyperparameters, potentially insufficient numbers of local training samples, and sometimes high local prediction errors. Also, implemented as an R package, GRF currently does not have a Python version which limits its adoption among machine learning practitioners who prefer Python. This work addresses these limitations by introducing theory-informed hyperparameter determination, local training sample expansion, and spatially weighted local prediction. We also develop a Python-based GRF model and package, PyGRF, to facilitate the use of the model. We evaluate the performance of PyGRF on an example dataset and further demonstrate its use in two case studies in public health and natural disasters.
Full paper link: https://doi.org/10.1111/tgis.13248
Preprint PDF: https://www.acsu.buffalo.edu/~yhu42/papers/2024_TGIS_PyGRF.pdf
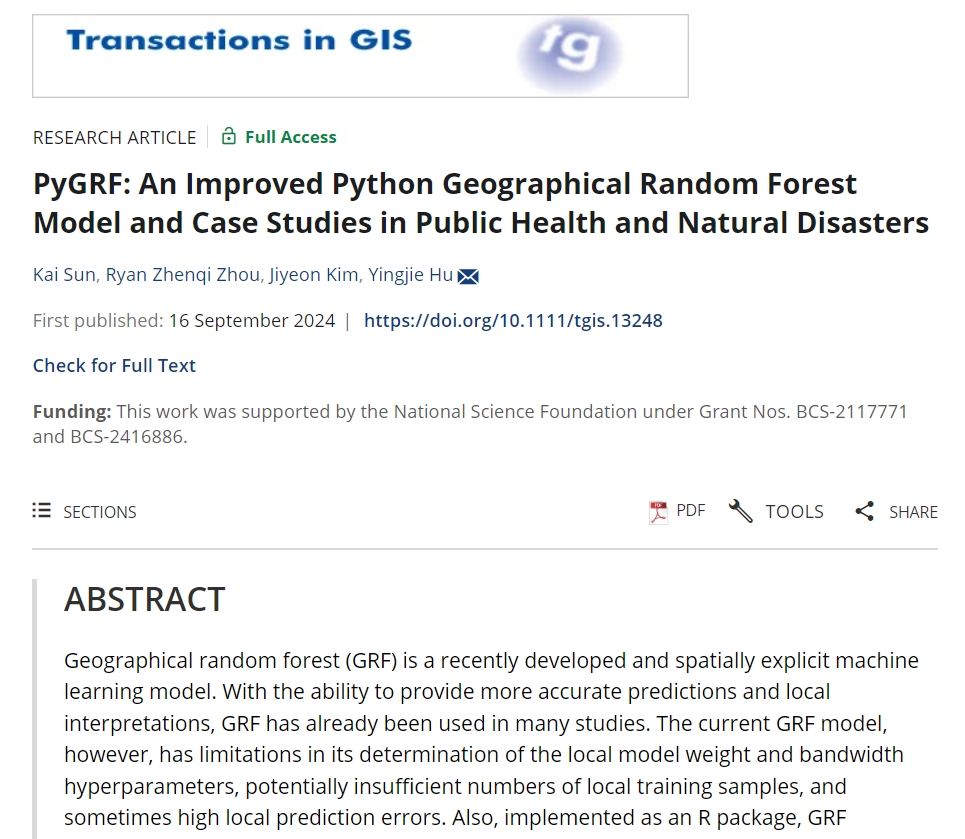