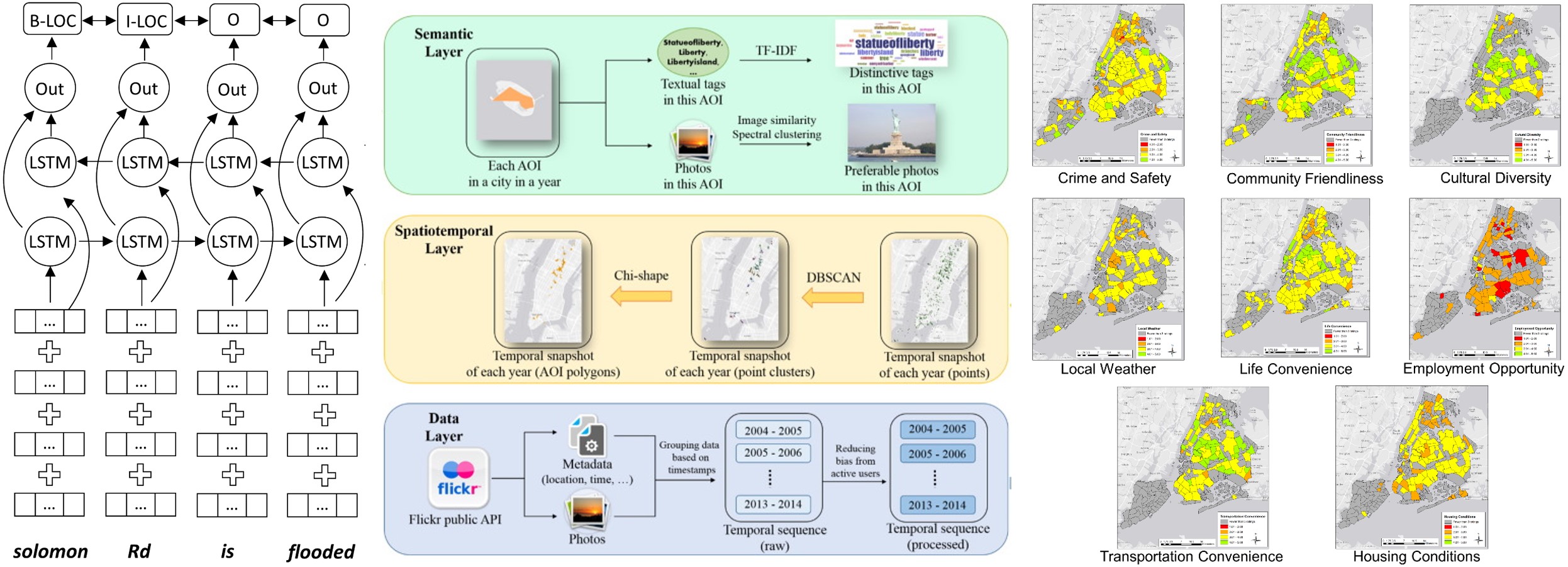
Geo-text and Information Retrieval: Our work in this area aims to develop methods and tools that can facilitate the retrieval and use of geo-text data. Many datasets nowadays contain links between geographic locations and texts (geo-text data for short). In addition, people nowadays often interact with smart devices through natural languages (e.g., Amazon’s Alexa), and there is an increasing demand for extracting spatial information from texts. Our research has produced computational methods for place name disambiguation and EUPEG: a unified platform for evaluating geoparsers. We also developed methods for active information retrieval based on information value theory to support daily tasks and prioritize information for disaster response.
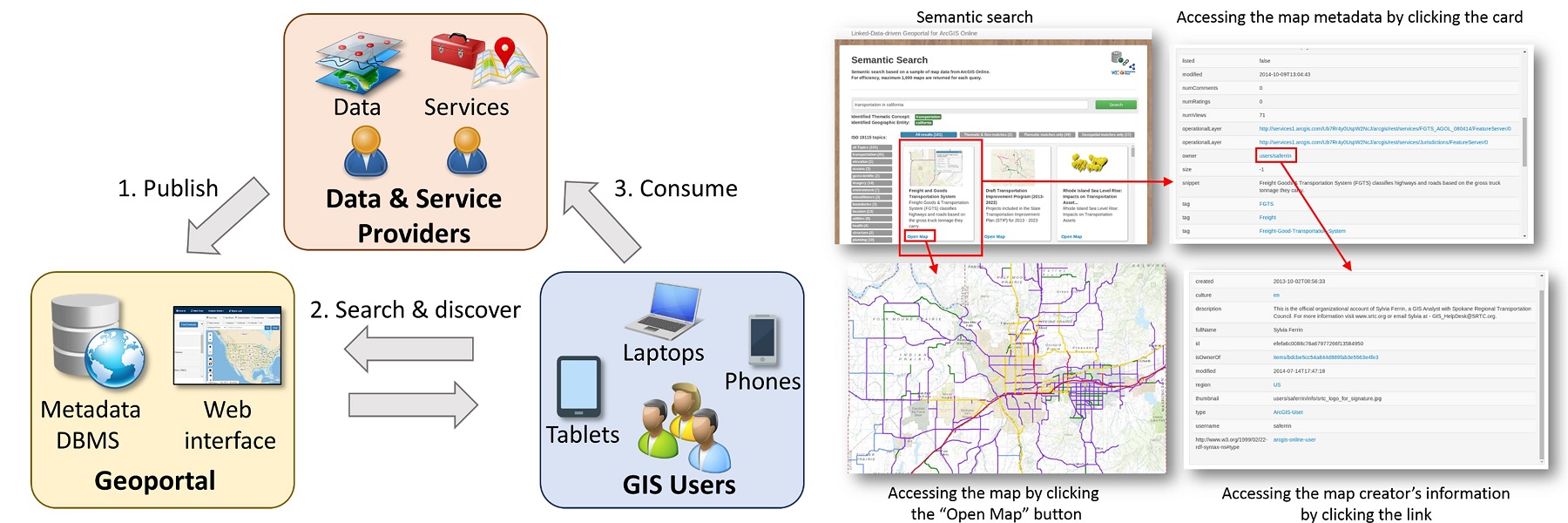