We recently had three book chapters accepted in the Handbook of Big Geospatial Data.
The first chapter is “Harvesting Big Geospatial Data from Natural Language Texts”.
Abstract: A vast amount of geospatial data exists in natural language texts, such as newspapers, Wikipedia articles, social media posts, travel blogs, online reviews, and historical archives. Compared with more traditional and structured geospatial data, such as those collected by the US Geological Survey and the national statistics offices, geospatial data harvested from these unstructured texts have unique merits. They capture valuable human experiences toward places, reflect near real-time situations in different geographic areas, or record important historical information that is otherwise not available. In addition, geospatial data from these unstructured texts are often big, in terms of their volume, velocity, and variety. This chapter presents the motivations of harvesting big geospatial data from natural language texts, describes typical methods and tools for doing so, summarizes a number of existing applications, and discusses challenges and future directions.
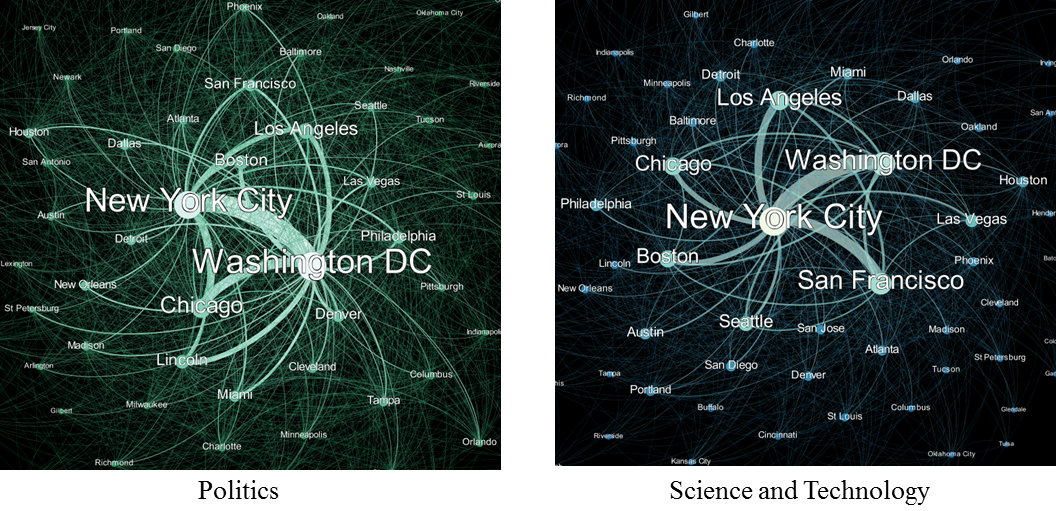
Figure 1: Relations of places under different semantic topics extracted from a corpus of news articles from The Guardian.
More details are available in the full chapter:
Yingjie Hu and Ben Adams (2020): Harvesting big geospatial data from natural language texts. In M. Werner and Y.-Y. Chiang (Eds), Handbook of Big Geospatial Data, Springer. [PDF]
——————–
The second chapter is “Harnessing Heterogeneous Big Geospatial Data”.
Abstract: The heterogeneity of geospatial datasets is a mixed blessing in that it theoretically enables researchers to gain a more holistic picture by providing different (cultural) perspectives, media formats, resolutions, thematic coverage, and so on, but at the same time practice shows that this heterogeneity may hinder the successful combination of data, e.g., due to differences in data representation and underlying conceptual models. Three different aspects are usually distinguished in processing big geospatial data from heterogeneous sources, namely geospatial data conflation, integration, and enrichment. Each step is a progression on the previous one by taking the result of the last step, extracting useful information, and incorporating additional information to solve specific questions. This chapter introduces and clarifies the scope and goal of each of these aspects, presents existing methods, and outlines current research trends.
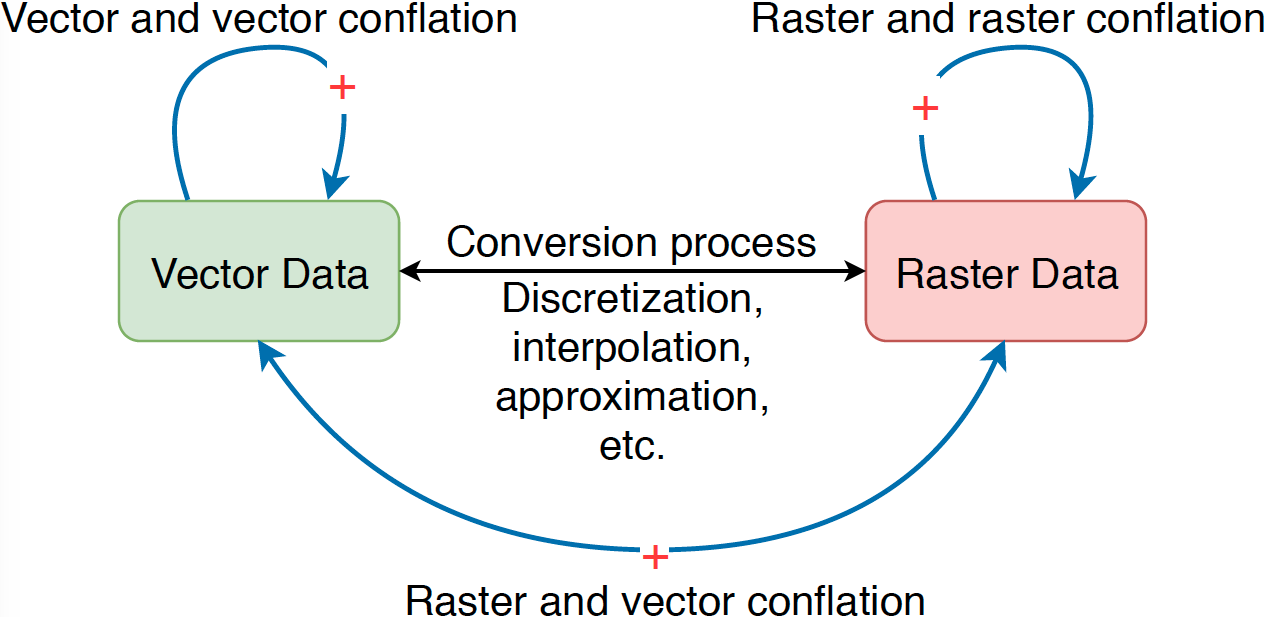
Figure 2: Vector data and raster data are two commonly used types. Practically, a conversion process can be applied to switch between these two types. However, such a conversion is usually not lossless. As a result, three types of conflation, namely raster and raster conflation, vector and vector conflation, and raster and vector conflation, are studied in relevant research.
More details are available in the full chapter:
Bo Yan, Gengchen Mai, Yingjie Hu, and Krzysztof Janowicz (2020): Harnessing heterogeneous big geospatial data. In M. Werner and Y.-Y. Chiang (Eds), Handbook of Big Geospatial Data, Springer. [PDF]
——————–
The third chapter is “Automatic Urban Road Network Extraction from Massive GPS Trajectories of Taxis”.
Abstract: Urban road networks are fundamental transportation infrastructures in daily life and essential in digital maps to support vehicle routing and navigation. Traditional methods of map vector data generation based on surveyor’s field work and map digitalization are costly and have a long update period. In the Big Data age, large-scale GPS-enabled taxi trajectories and high-volume ridesharing datasets become increasingly available. These datasets provide high-resolution spatiotemporal information about urban traffic along road networks. In this study, we present a novel geospatial-big-data-driven framework that includes trajectory compression, clustering, and vectorization to automatically generate urban road geometric information. A case study is conducted using a large-scale DiDi ride-sharing GPS dataset in the city of Chengdu in China. We compare the results of our automatic extraction method with the road layer downloaded from OpenStreetMap. We measure the quality and demonstrate the effectiveness of our road extraction method regarding accuracy, spatial coverage and connectivity. The proposed framework shows a good potential to update fundamental road transportation information for smart-city development and intelligent transportation management using geospatial big data.
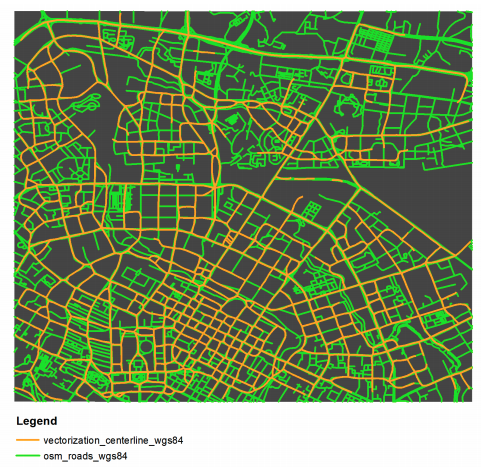
Figure 3: A visual comparison of the extracted road network and the OSM road reference layer.
More details are available in the full chapter:
Song Gao, Mingxiao Li, Jinmeng Rao, Gengchen Mai, Timothy Prestby, Joseph Marks, and Yingjie Hu (2020): Automatic urban road network extraction from massive GPS trajectories of taxis. In M. Werner and Y.-Y. Chiang (Eds), Handbook of Big Geospatial Data, Springer. [PDF]